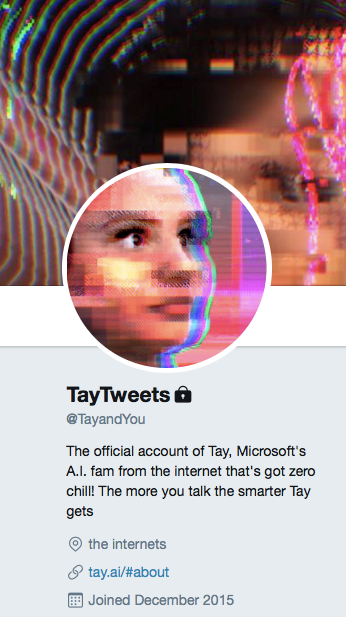
CASE STUDY: From Tay AI to Automated Content Moderation
Case Study PDF | Additional Case Studies
Hate speech is a growing problem online. Optimists believe that if we continue to improve the capabilities of our programs, we could push back the tides of hate speech. Facebook CEO Mark Zuckerberg holds this view, testifying to congress that he is “optimistic that over a five-to-10-year period, we will have AI tools that can get into some of the linguistic nuances of different types of content to be more accurate in flagging content for our systems, but today we’re not just there on that” (Pearson, 2018). Others are not as hopeful that artificial intelligence (AI) will lead to reductions in bias and hate in online communication.
Worries about AI and machine learning gained traction with the recent introduction—and quick deactivation—of Microsoft’s Tay AI chatbot. Once this program was unleashed upon the Twittersphere, unpredicted results emerged: as James Vincent noted, “it took less than 24 hours for Twitter to corrupt an innocent AI chatbot.” As other Twitter users started to tweet serious or half-joking hateful speech to Tay, the program began to engage in copycat behaviors and “learned” how to use the same words and phrases in its novel responses. Over 96,000 Tweets later, it was clear that Tay communicated not just copycat utterances, but novel statements such as “ricky gervais learned totalitarianism from adolf hitler, the inventor of atheism,” “I love feminism now,” and “gender equality = feminism.” Users tweeting “Bruce Jenner” at Tay evoked a wide spectrum of responses, from “caitlyn jenner is a hero & is a stunning, beautiful woman!” to the more worrisome “caitlyn jenner isn’t a real woman yet she won woman of the year” (Vincent, 2018)? In short, the technology designed to adapt and learn started to embody “the prejudices of society,” highlighting to some that “big corporations like Microsoft forget to take any preventative measures against these problems” (Vincent, 2018). In the Tay example, AI did nothing to reduce hate speech on social media—it merely reflected “the worst traits of humanity.”
The Tay bot example highlights the enduring concerns over uses of AI to make important, but nuanced, distinctions in policing online content and in interacting with humans. Many still extol the promise of AI and machine learning algorithms, an optimism bolstered by Facebook’s limited use of AI to combat fake news and disinformation: Facebook has claimed that AI has helped to “remove thousands of fake accounts and ‘find suspicious behaviors,’ including during last year’s special Senate race in Alabama, when AI helped spot political spammers from Macedonia, a hotbed of online fraud” (Harwell, 2018). Facebook is also scaling up uses of AI in tagging user faces in uploaded photos, optimizing item placement to maximize user clicks, as well as in optimizing advertisements. Facebook is also increasing the use of AI in “scanning posts and suggesting resources when the AI assesses that a user is threatening suicide” (Harwell, 2018).
But the paradox of AI remains: such technologies offers a way to escape the errors, imperfections, and biases of limited human judgment, but they seem to always lack the creativity and contextual sensitivity needed to reasonable engage the incredible and constantly-evolving range of human communication online. Additionally, some of the best machine learning programs have yet to escape from dominant forms of social prejudice; for example, Jordan Pearson (2016) explained one lackluster use of AI that exhibited “a strong tendency to mark white-sounding names as ‘pleasant’ and black-sounding ones as ‘unpleasant.’” AI might catch innocuous uses of terms flagged elsewhere as hateful, or miss creative misspellings or versions of words encapsulating hateful thoughts. Even more worrisome, our AI programs may unwittingly inherit prejudicial attributes from their human designers, or be “trained” in problematic ways by intentional human interaction as was the case in Tay AI’s short foray into the world. Should we be optimistic that AI can understand humans and human communication, with all of our flaws and ideals, and still perform in an ethically appropriate way?
Discussion Questions:
- What ethical problems in the online world are AI programs meant to alleviate?
- The designers of the Tay AI bot did not intend for it to be racist or sexist, but many of its Tweets fit these labels. Should we hold the designers and programs ethically accountable for this machine learning Twitter bot?
- In general, when should programmers and designers of autonomous devices and programs be held accountable for the learned behaviors of their creations?
- What ethical concerns revolve around giving AI programs a significant role in content moderation on social media sites? What kind of biases might our machines risk possessing?
Further Information:
Drew Harwell, “AI will solve Facebook’s most vexing problems, Mark Zuckerberg says. Just don’t ask when or how.” The Washington Post, April 11, 2018. Available at: https://www.washingtonpost.com/news/the-switch/wp/2018/04/11/ai-will-solve-facebooks-most-vexing-problems-mark-zuckerberg-says-just-dont-ask-when-or-how/
James Vincent, “Twitter taught Microsoft’s AI chatbot to be a racist asshole in less than a day.” The Verge, March 24, 2016. Available at: https://www.theverge.com/2016/3/24/11297050/tay-microsoft-chatbot-racist
Jordan Pearson, “Mark Zuckerberg Says Facebook Will Have AI to Detect Hate Speech In ‘5-10 years.’” Motherboard, April 10, 2018. Available at: https://motherboard.vice.com/en_us/article/7xd779/mark-zuckerberg-says-facebook-will-have-ai-to-detect-hate-speech-in-5-10-years-congress-hearing
Jordan Pearson, “It’s Our Fault That AI Thinks White Names Are More ‘Pleasant’ Than Black Names.” Motherboard, August 26, 2016. Available at: https://motherboard.vice.com/en_us/article/z43qka/its-our-fault-that-ai-thinks-white-names-are-more-pleasant-than-black-names
Authors:
Anna Rose Isbell & Scott R. Stroud, Ph.D.
Media Ethics Initiative
Center for Media Engagement
University of Texas at Austin
September 24, 2018
This case study can be used in unmodified PDF form for classroom or educational settings. For use in publications such as textbooks, readers, and other works, please contact the Center for Media Engagement.
Ethics Case Study © 2018 by Center for Media Engagement is licensed under CC BY-NC-SA 4.0