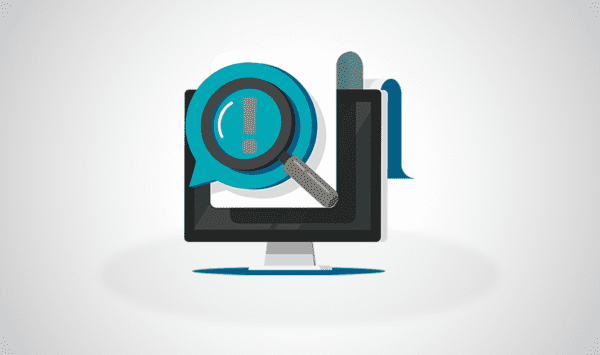
SUMMARY
To find out how people in various countries feel about social media platforms and news organizations deleting offensive comments, the Center for Media Engagement teamed up with researchers in the Netherlands and Portugal. The study looked at three aspects of comment deletion: whether a human moderator or an algorithm deleted the content, the type of deleted content (profanity or hate speech), and the level of detail in the explanation for the deletion.
The findings suggest that social media platforms and newsrooms consider the following when deleting comments:
- Moderators should focus more on hate speech, because people see hate speech as more in need of deletion than profanity.
- Moderators should explain specifically why content was removed, rather than offer general explanations.
- Algorithmic moderators may be perceived equally to human moderators, although specific cultural contexts should be considered because this may not be the case in every country.
THE PROBLEM
Social media platforms and news organizations often delete comments that are offensive as a means to improve online discussions.1 In this project, the Center for Media Engagement teamed up with researchers from Erasmus University in the Netherlands and NOVA University in Portugal to examine how the public perceives comment deletion and the moderators who do it.
We looked at three aspects of comment deletion. We first considered whether a human moderator or an algorithm deleted the comment. Use of algorithms or other forms of artificial intelligence are increasingly seen as a solution2 for comment moderation because, as a Center for Media Engagement study found, the task is emotionally exhausting for humans.3 We also considered whether the type of deleted content (profanity or hate speech) or the level of detail explaining the deletion influenced people’s perceptions about the deletion or the moderator who did it. This project was funded by Facebook. All research was conducted independently.
KEY FINDINGS
- Across all three countries, people perceived the deletion of hate speech as more fair and legitimate than the removal of profanity. They also perceived moderators who removed hate speech as being more transparent. U.S. and Dutch participants perceived moderators who removed hate speech as more trustworthy than those who removed profanity, although this was not the case in Portugal.
- The type of moderator – human or an algorithm – had no effect on people’s perceptions about the deletion of content in the U.S. or the Netherlands. In Portugal, people perceived deletions by human moderators as more fair and legitimate compared to deletions by algorithms.
- People in all three countries felt the platform was being more transparent if it explained in detail why the content was removed. However, the extent of detail explaining the deletion had no effect on perceptions of how fair or legitimate the deletion was or whether people perceived the moderator as trustworthy.
IMPLICATIONS
The findings offer some clear takeaways for social media platforms and news organizations regarding comment deletion:
- Moderators should focus more on hate speech, because people see hate speech as more in need of deletion than profanity.
- Moderators should explain specifically why content was removed, rather than offer general explanations.
- Algorithmic moderators may be perceived equally to human moderators, although specific cultural contexts should be considered because this may not be the case in every country.
FULL FINDINGS
Participants were exposed to a social media post that contained either hate speech or profanity. Then they were exposed to a post from a moderator – either a human or an algorithm – that deleted the initial post because it was offensive. This message either explained specifically why the post was deleted, gave a general sense of why it was deleted with a clickable link to community guidelines for the site, or offered no explanation. Afterward, participants answered questions about how fair4 or legitimate5 the deletion was and how transparent6 or trustworthy7 the moderator was.
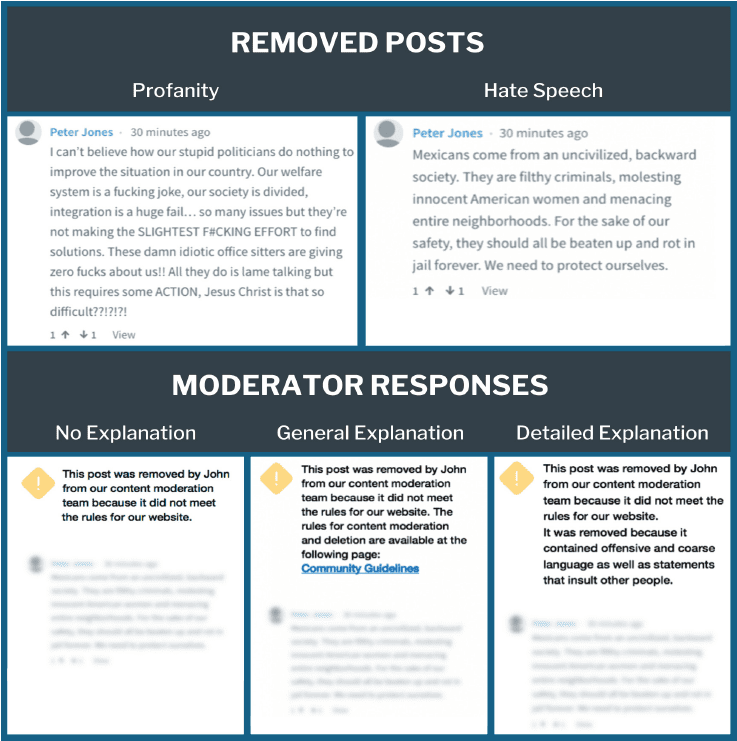
In the U.S. and the Netherlands, people perceived the deletion of comments equally in every case, regardless of whether a human or an algorithm was the moderator.8 In contrast, in Portugal, participants saw deletion of comments as more fair and legitimate if it was done by a human, rather than an algorithm.9
Across all three countries, participants perceived the deletion of hate speech as more fair10 and legitimate11 than the deletion of profanity. Posts with profanity contained swear words, while hate speech posts targeted vulnerable groups with discriminatory statements.12
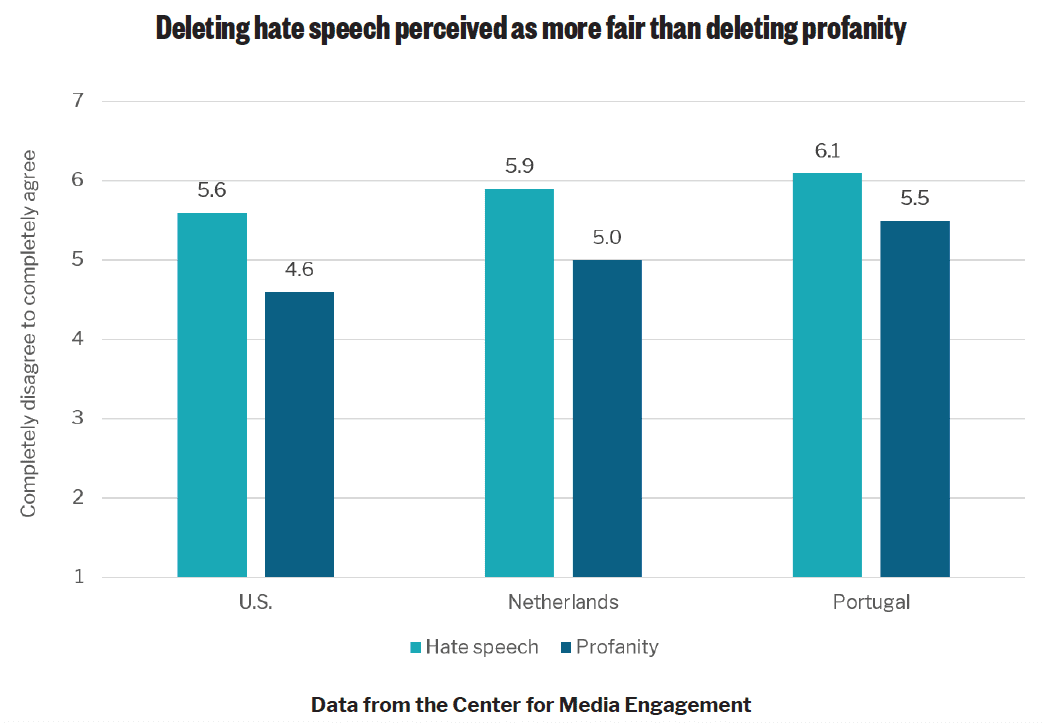
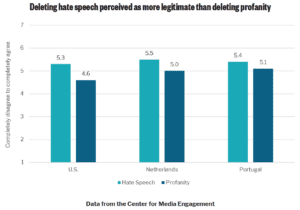
Next, we considered how people perceived the moderator. Across all three countries, people perceived the moderator as more transparent13 if the content that was deleted was hate speech, rather than profanity, and if a more detailed explanation for the deletion was given. The detailed explanation noted why the content was removed. This was compared to messages that explained that the content was removed for violating the platform’s rules without specifying which rules (no explanation) or that directed participants to the platform’s guidelines with no specific explanation for why the content was deleted (general explanation).
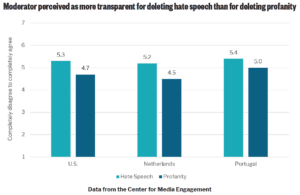
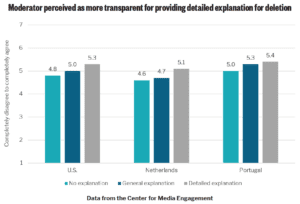
Finally, we considered how people assessed the trustworthiness of the moderator who had deleted the content. Across the U.S. and the Netherlands, the moderator was considered most trustworthy for deleting hate speech as compared with deleting profanity, but no significant difference was found in Portugal.14
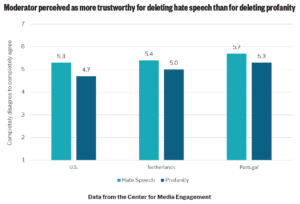
METHODOLOGY
Participants (N = 2,870)15 were recruited using Dynata to create samples that mirrored the demographics of each country.16 A total of 902 people participated in the United States, 975 in the Netherlands, and 993 in Portugal. All materials were translated into Dutch and Portuguese for participants in the Netherlands and Portugal by research team members fluent in all three languages.
Participants were randomly assigned to see a social media post that contained either profanity or hate speech.17 The post was designed to resemble the online discussion template Disqus because Disqus is used in diverse discussion spaces and is not limited to one platform, like Facebook or Twitter.
Participants then saw a follow-up message stating that the initial post had been removed, along with a blurred version of the original post. They were randomly assigned to either receive this message from a human moderator or from an algorithm. They were additionally randomly assigned to see one of three messages with varying levels of explanation about why the content was removed. One message stated the content was removed because it violated the platform’s rules (no explanation), one message directed the user to a link to the actual community guidelines but did not explain why the content was removed (general explanation), and one message disclosed the specific reason by detailing why the content was removed (detailed explanation).18
After being shown this second message, participants answered questions about how fair and legitimate the act of deletion was and how transparent and trustworthy they felt the moderator who did the deletion was.
Suggested Citation
Masullo, Gina M., Gonçalves, João, Weber, Ina, Laban, Aquina, Torres da Silva, Marisa, and Hofhuis, Joep. (June, 2021). How the public views deletion of offensive comments. Center for Media Engagement. https://mediaengagement.org/research/how-the-public-views-deletion-of-offensive-comments
- Boberg, S., Schatto-Eckrodt, T., Frischlich, L, & Quant, T. (2018). The moral gatekeeper? Moderation and deletion of user-generated content in a leading news forum. Media and Communication, 6(4), 58-69; Ksiazek, T. B. (2015). Civil interactivity: How news organizations’ commenting policies explain civility and hostility in user comments. Journal of Broadcasting & Electronic Media, 59(4), 556–573. [↩]
- Gillespie, T. (2020). Content moderation, AI, and the question of scale. Big Data & Society, 7(2), 1-5. [↩]
- Riedl, M. J., Chen, G.M., & Whipple, K. N. (2019, July). Moderating uncivil comments hurts trust in news. Center for Media Engagement. https://mediaengagement.org/research/moderating-uncivil-comments [↩]
- Fairness was measured by ratings on a 1 (completely disagree) to 7 (completely agree) scale for nine statements from Colquitt, J.A. (2001). On the dimensionality of organizational justice: A construct validation of a measure. Journal of Applied Psychology 86(3): 386–400. These were: “The moderator’s decision is appropriate in regard to the post,” “The removal of the post is justified,” “I am satisfied with the removal of the post,” “I think it was fair to remove the post,” “This moderation process has followed ethical and moral standards,” “The moderator has treated the author of the post with respect,” “The moderator’s explanation of the moderation process is reasonable,” “The moderation of this post was executed in a fair way,” and “The moderator’s way of handling this post was proper.” A principal component analysis with promax rotation showed these loaded on one factor, so they were averaged into an index for each country, Cronbach’s α = USA0.96, Neth0.97, and Por0.95. [↩]
- Legitimacy was measured by ratings on a 1 (completely disagree) to 7 (completely agree) scale for five statements from van der Toorn J., Tyler, T.R., & Jost, J.T. (2011). More than fair: Outcome dependence, system justification, and the perceived legitimacy of authority figures. Journal of Experimental Social Psychology, 47(1): 127-138. These were: “This moderator is a legitimate authority and users should follow their decisions,” “I accept the moderator’s judgment of this post without questioning it,” “The moderator has the right to evaluate posts that users make on this social networking site,” “You should accept the removal of a post even when you disagree with it,” and “You should accept the removal of a post even when you don’t understand the reasons for the moderator’s decision.” These were averaged together into an index for each country, Cronbach’s α = USA0.89, Neth0.86, and Por0.80. [↩]
- Transparency was measured using ratings on a 1 (completely disagree) to 7 (completely agree) scale for five statements adapted from Rawlins, B. (2008). Measuring the relationship between organizational transparency and employee trust. Public Relations Journal, 2(2): 1–21. These were: “I was provided with information that is useful to understand the removal of the post,” “I was provided with detailed information to understand the removal of the post,” “I was provided with information that is relevant to understand the removal of the post,” “I was provided with information about the removal of the post that is easy to understand,” and “I was provided with complete information about the removal of the post.” These were averaged into an index for each country, Cronbach’s α = USA0.95, Neth0.95, and Por0.94. [↩]
- Trust was measured using ratings on a 1 (completely disagree) to 7 (completely agree) scale from Rawlins, 2008. These were: “I trust the moderator to be interested in the well-being of the users like me on this social networking site,” “I feel very confident about the competencies of the moderator when making decisions about posts on this social networking site,” “I think the moderator is reliable when it comes to deciding which posts to remove,” “I think the moderator is honest when evaluating posts on this social networking site,” and “The moderator was impartial when deciding to remove the post.” These were averaged into an index for each country, Cronbach’s α = USA0.93, Neth0.91, and Por0.89. [↩]
- All the findings were tested by a series of multi-factorial ANOVAs, one in each country for each dependent variable. In each analysis, message type (hate speech versus profanity), moderator type (human versus algorithm), and message content (no explanation for comment deletion, general explanation, and detailed explanation) were entered as between-subjects’ factors. Initially, interactions between attitudes toward the comment were tested as an interaction with each independent variable. Only Portugal produced significant interactions for message type and agreement with the comment and for moderator type and agreement with the comment when trustworthiness of the moderator was the dependent variable, so models including the interactions are reported. For all other analyses, interactions were dropped from the final models reported here. [↩]
- In Portugal, the average score for fairness of deletion was 5.9 for a human moderator, compared to 5.6 for an algorithm (p = .003), and the average score for legitimacy of deletion was 5.3 for a human moderator, compared to 5.1 for an algorithm (p = .01). [↩]
- For perception that the deletion was fair, a main effect was found for message type in the U.S., {F (1, 897) = 94.79, p < .001, η2 = 0.09}; the Netherlands, {F (1, 970) = 106.57, p < .001, η2 = 0.10}; and Portugal, {F (1, 988) =66.56, p < .001, η2 = 0.06}. Portugal also showed a main effect for moderator type, {F (1, 988) = 8.70, p = .003, η2= 0.001}, but the U.S. or the Netherlands did not. No main effects were found for message content in any of the countries. [↩]
- For perception that the deletion was legitimate, a main effect was found for message type in the U.S., {F (1, 897)= 49.21, p < .001, η2 = 0.05}; the Netherlands {F (1, 969) = 42.48, p < .001, η2 = 0.04}; and Portugal, {F (1, 988) =14.05, p < .001, η2 = 0.01}. Portugal also showed a main effect for moderator type, {F (1, 988) = 7.704, p = .01, η2= 0.001}, but the U.S. and the Netherlands did not. No main effects were found for message content in any of the countries. [↩]
- We based these categories on definitions of hateful speech from ECRI. (2016). ECRI General Policy Recommendation 15 on combating hate speech. Strasbourg: Council of Europe; Article 19. (2015). Hate speech explained. A toolkit: London; Gagliardone, I., Gal, D., Alves, T., & Martinez, G. (2015). Countering online hate speech. Paris: UNESCO. Our profanity condition was based on the conceptualization of incivility from Chen, G.M. (2017). Online incivility and public debate: Nasty talk. Palgrave Macmillan, and Muddiman, A. (2017). Personal and public levels of political incivility. International Journal of Communication, 11, 3182–3202. [↩]
- For perception that the moderator was transparent, a main effect was found for message type in the U.S., {F (1, 897) = 41.94, p < .001, η2 = 0.04}, the Netherlands, {F (1, 969) = 44.84, p < .001, η2 = 0.04}; and Portugal, {F (1, 987) = 24.38, p < .001, η2 = 0.02}. A main effect was also found for message content in the U.S., {F (2, 896) = 9.14, p < .001, η2 = 0.02}; the Netherlands, {F (2, 969) = 41.94, p < .001, η2 = 0.04}; and Portugal, {F (2, 987) = 9.87, p < .001, η2 = 0.02}. No significant effects were found for moderator type. [↩]
- For perception that the moderator was trustworthy, a main effect was found for message type in the U.S., {F (1, 890) = 34.71, p < .001, η2 = 0.04} and the Netherlands, {F (1, 956) = 41.18, p < .001, η2 = 0.04} but not in Portugal. No main effects were found for message content or moderator type in any of the countries. [↩]
- Erasmus University in the Netherlands granted Ethics Review Board approval for the project on February 3, 2020. The University of Texas at Austin’s Institutional Review Board approved the project on February 26, 2020. Initially, 4,636 people participated, but data were not analyzed for those who did not answer a question testing if they were paying attention (n = 648), those who answered that question incorrectly (n = 878), those who sped through the questions (n = 213), and those who seemingly answered the questions without reading them carefully (n = 27). This resulted in 2,870 participants. [↩]
- Matches were based on age, gender, and education and on race/ethnicity in some cases. Race/ethnicity questions were not asked of the Portuguese because these are prohibited by the Portuguese Constitution. In the Netherlands, the country of birth of the mother/father were asked as proxy for race and ethnicity, as U.S. categories do not translate well to this context. For the U.S. samples, Dynata matched demographics of the U.S. adult internet population based on a random sample survey conducted by Pew Research Center. [↩]
- Before the experiment, a pretest (n = 304) was conducted in the three countries to ensure that people were interpreting profanity and hate speech messages in similar ways. First, 10 messages (five profane and five hate speech) were created, using the definitions of these concepts explained in endnote 12. Then pretest respondents were asked the extent to which each message contained profanity or hate speech, and the messages rated most profane or most hateful were selected for the experiment. The message selected for the profanity condition was rated as significantly (p < .001) more profane (M = 3.95) than the message for the hate speech condition (M = 3.12). Likewise, participants reported a significantly (p < .001) higher presence of hate speech for the message in the hate speech condition (M = 4.35) than for the message in the profanity condition (M = 3.66). Messages were translated and adapted to their respective contexts. For instance, the hate speech message targeted Mexicans in the U.S., Brazilians in Portugal, and foreigners in the Netherlands. For a more in-depth description of the pretest, see: Weber, I., Laban, A., Masullo, G.M., Gonçalves, J., Torres da Silva, M., & Hofhuis, J. (2020, September). International perspectives on what’s considered hateful or profane online. Center for Media Engagement. https://mediaengagement.org/research/perspectives-on-online-profanity [↩]
- Random assignment was successful, with no significant differences across conditions in terms of age, gender, race/ethnicity, or education. Manipulation checks also indicate that our manipulations of moderator type X2 (3, N=2,817) = 832.25, p < .001 and message type X2 (4, N=2,739) = 519.68, p < .001 were successful. [↩]